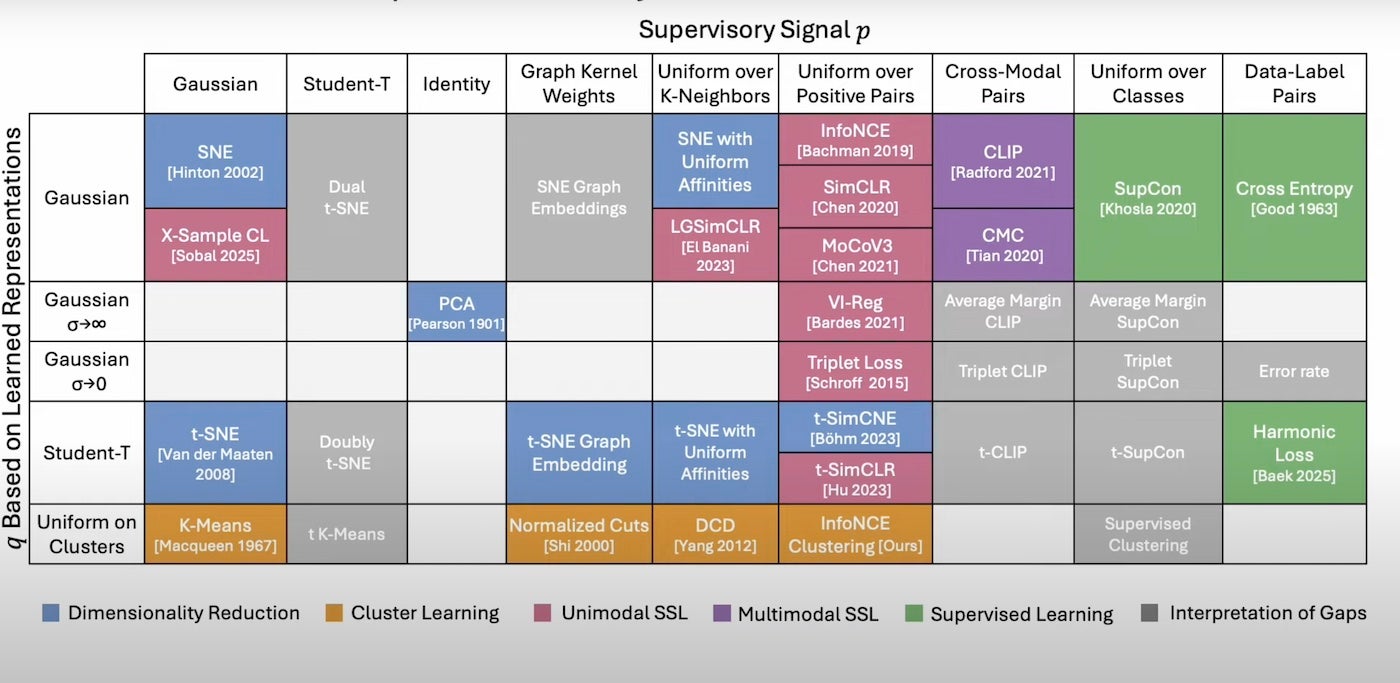
A new “periodic table for machine learning,” is reshaping how researchers explore AI, unlocking fresh pathways for discovery. The framework, Information-Contrastive Learning (I-Con), connects diverse machine learning (ML) methods, offering researchers a structured system for innovation.
Developed by researchers from MIT, Microsoft, and Google, I-Con not only provides a new lens for understanding ML but also encourages creative thinking by merging traditional techniques. This groundbreaking approach is poised to propel the field forward, opening up new research possibilities and establishing clearer directions for future advancements.
How the I-Con framework unlocks new research paths
The I-Con framework opens new avenues for AI discovery by organizing more than 20 ML algorithms into a unified structure, much like a periodic table for machine learning.
“We’re starting to see machine learning as a system with structure that is a space we can explore rather than just guess our way through,” said Shaden Alshammari, an MIT graduate student and lead author of the paper, introducing I-Con.
This structure is grounded in a single information-theoretic principle that captures core ideas across clustering, supervised learning, contrastive learning, and more. By making these hidden connections explicit, I-Con helps researchers spot overlooked relationships between methods and inspires the design of hybrid models that boost performance.
The framework boosts image classification by 8%
One breakthrough credited to I-Con involves fusing two previously unconnected algorithms to develop a new image-classification technique — outperforming state-of-the-art models on ImageNet-1K by 8 percent. And the framework doesn’t stop at known approaches: Like a true periodic table, it hints at missing elements, guiding researchers toward unexplored algorithmic combinations.
By consolidating machine learning approaches into a coherent map, I-Con is transforming how researchers think about model design — speeding up innovation and revealing fresh paths in the AI frontier.
DOWNLOAD this Machine Learning Quick Glossary from TechRepublic Premium
A roadmap for future AI innovation
Systematic exploration is becoming indispensable as machine learning moves from experimentation to real-world application, and I-Con delivers just that. With years of machine learning knowledge organized in a structured path, AI researchers are now well-equipped to quickly find promising algorithm combinations, accelerating the pace of discovery.
As AI tools become foundational across enterprises, I-Con brings researchers a smarter way to build more adaptable systems. Its ability to highlight gaps as well as mix and match proven techniques raises performance and leads to solutions that were previously unimaginable.
With systems like I-Con spearheading progress, the next era of AI is set to be more integrated and synergistic.